Optimizing Clinical Trials With Effective Data Management
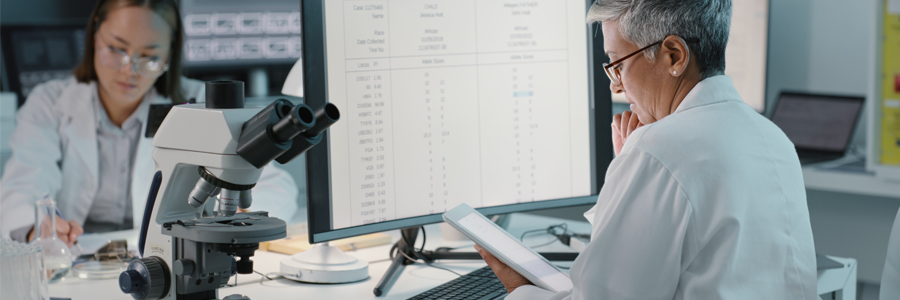
Clinical trials play a crucial role in advancing medical research and improving patient care. However, these trials are often complex and resource-intensive, requiring careful management of vast amounts of data.
In this article, we will explore the importance of effective data management in optimizing clinical trials. We will discuss the challenges faced by researchers in handling data, such as data quality issues, regulatory compliance and the need for real-time access to accurate information. Additionally, we will go through the benefits of implementing robust data management strategies, including improved efficiency, enhanced data integrity and accelerated decision-making.
What is clinical trials data?
Clinical trials data refers to the information collected during research studies conducted on human subjects to evaluate the safety and effectiveness of new medical interventions and treatments. It encompasses a wide range of data points, including patient demographics, medical history, laboratory test results, treatment outcomes and adverse events. Clinical trials data is important in advancing medical knowledge and shaping evidence-based healthcare practices.
Importance of Clincial Trials Data
Clinical trials data holds immense importance in the field of medical research and drug development. It serves as a valuable resource for researchers, biostatisticians and regulatory authorities, aiding in the evaluation of treatment efficacy and safety. The insights drawn from clinical trials data help inform decision-making at various stages of drug development, from protocol design to market entry.
One of the key beneficiaries of clinical trials data is the biostatistician. They rely on this data to analyze and interpret the outcomes of clinical trials, assessing the statistical significance of treatment effects and potential side effects. By examining the data, biostatisticians can make informed and insightful decisions regarding drug development protocols. They can identify patterns, trends and correlations within the data, enabling them to propose necessary changes to upcoming clinical trial stages to enhance the study’s efficacy and validity.
Moreover, clinical trials data can help expedite the availability of new drugs in the market. Regulatory authorities, such as drug regulatory agencies, heavily rely on robust clinical trials data to evaluate the safety and effectiveness of a new intervention. The data provides crucial evidence to support the submission of regulatory applications and allows authorities to make informed decisions regarding drug approvals. By providing comprehensive and reliable data, clinical trials accelerate the regulatory review process and help bring life-enhancing and life-saving medications to patients sooner.
What Components Make Up Clinical Trials Data?
The components of clinical trials data encompass a wide range of metrics and information that are crucial for evaluating treatment efficacy, safety and patient outcomes. By compiling and analyzing these components, clinical trials generate valuable data that provides evidence for regulatory authorities, healthcare professionals and researchers to make informed decisions about the safety and efficacy of medical interventions.
Patient Demographics
Patient demographics encompass essential information, such as age, gender, race and ethnicity. These metrics provide insights into how different demographic factors may influence treatment responses, potential disparities in healthcare outcomes and the generalizability of study results across diverse populations.
Medical History
The medical history component involves collecting data on preexisting medical conditions, prior treatments, surgeries and medication usage. Understanding a participant’s medical history allows researchers to assess the impact of these factors on treatment outcomes, potential interactions with the investigational intervention and general health status of the study population.
Laboratory Test Results
Laboratory test results play a crucial role in clinical trials. These tests involve analyzing samples collected from participants and can include blood, plasma, urine, tissues, feces and cells, among others. Metrics measured in the laboratory data component may include biomarkers, disease-specific indicators, physiological parameters and other relevant measurements. These measurements provide valuable insights into treatment effects, disease progression, potential adverse events and biochemical changes related to the study objectives.
Treatment-Specific Metrics
Treatment-specific metrics vary depending on the nature of the intervention being studied. In drug trials, metrics measured may include dosage levels, administration routes, treatment duration and adherence to the prescribed regimen. In surgical or medical device trials, metrics may include details about the specific procedures performed, device settings or technical parameters. These metrics help researchers understand the specific characteristics and application of the intervention under investigation.
Adverse Events Reporting
Adverse events reporting is a critical component of clinical trials data. It involves the systematic collection of information regarding any unexpected or undesirable reactions, side effects or complications experienced by participants during the study. Metrics collected in adverse events reporting may include the type, severity, duration and frequency of adverse events. This information helps researchers assess the safety profile of the intervention, determine its risk-benefit ratio and make informed decisions about its continued use.
Patient-Reported Outcomes
Patient-reported outcomes (PROs) capture subjective data directly reported by study participants. These metrics can include measures of symptom severity, quality of life, pain scores, functional assessments or other relevant self-reported information. Patient-reported outcomes provide insights into the participants’ subjective experiences and perspectives on treatment effectiveness, overall well-being and impact of the intervention on their daily lives.
Data Management Processes
Data management processes are an integral part of clinical trials. They encompass various activities, such as data collection, storage, analysis and reporting. Effective data management ensures the integrity, accuracy and privacy of clinical trials data. It involves implementing standardized data collection protocols, using secure systems for data storage, performing rigorous data analysis and generating comprehensive reports to support the study findings and regulatory submissions.
What is clinical data management?
Clinical data management refers to the systematic and controlled process of collecting, organizing, verifying and storing data generated during clinical trials or other healthcare-related studies. It involves ensuring the accuracy, completeness, consistency and integrity of the data throughout the entire data life cycle, from data capture to final analysis and reporting. The primary goal of clinical data management is to produce high-quality, reliable and interpretable data that can be used for meaningful analysis, decision-making and regulatory submissions.
Clinical data management professionals play a crucial role in ensuring that data is collected in a standardized and reliable manner, following predefined protocols and guidelines. They employ specialized tools and technologies to manage and track data, ensuring its quality, traceability and compliance with regulatory requirements.
Importance of Proper Data Management in Clinical Trials
Proper data management is of paramount importance in clinical trials for several reasons. First, it ensures the integrity and reliability of the data collected during the study. By implementing standardized data collection processes, data management professionals minimize errors, inconsistencies and missing data, thus improving the overall quality and accuracy of the data set.
Accurate data management also facilitates efficient data analysis. Researchers heavily rely on well-organized and properly documented data to perform meaningful statistical analyses, identify trends and draw valid conclusions. A robust data management system enables the integration and harmonization of data from different sources, allowing researchers to conduct comprehensive analyses and generate reliable insights.
Additionally, proper data management in clinical trials allows the researchers to ensure regulatory compliance. Regulatory authorities require comprehensive and accurate data to evaluate the safety and effectiveness of new interventions. By adhering to rigorous data management practices, clinical trials can provide complete and reliable data for regulatory submissions, expediting the review process and facilitating the approval of potentially life-enhancing and life-saving treatments.
The data collection process
The data collection process involves gathering and documenting various types of information to generate meaningful insights and draw valid conclusions. Effective data management plays a pivotal role in ensuring the accuracy, reliability and integrity of the collected data.
During the data collection process, researchers employ various methodologies to capture relevant information. This may include conducting interviews, administering surveys, performing physical examinations, utilizing medical devices or analyzing biological samples. The specific methods used depend on the nature of the study and the data required to address the research objectives.
Best practices for collecting accurate and complete data
Collecting accurate and complete data is paramount when conducting a clinical trial, as it ensures the validity and reliability of the study’s findings. To achieve this, researchers must follow best practices that emphasize the importance of accuracy and completeness.
Neglecting the importance of collecting accurate and complete data can lead to severe consequences. Researchers may unintentionally arrive at misleading or biased conclusions, impeding scientific progress and hindering their ability to secure funding for future studies. Patients may be subjected to ineffective or inappropriate treatments, posing risks to their well-being and overall health.
Additionally, hospitals and institutions may suffer damage to their reputation due to compromised data. Therefore, it’s essential to implement these best practices to minimize errors and enhance data quality and reliability.
Standardized Data Collection Procedures
Implementing standardized procedures for data collection is vital to ensure consistency across different study sites and reduce variability in data. The standardized data collection processes commonly followed in research studies include:
Development of Study Protocol: Researchers create a detailed protocol that outlines the study objectives, research questions and specific data to be collected. This serves as a guide throughout the data collection process.
Clear Data Collection Procedures: Precise instructions and procedures are established to ensure uniform and accurate data collection. These guidelines specify the methods, tools and techniques to be used, including electronic data collection methods such as electronic patient-reported outcomes (ePRO) and connected devices.
Training for Data Collectors: Data collectors receive comprehensive training on the study protocol, data collection procedures and relevant instruments or tools, including training on electronic data collection technologies. This ensures consistent understanding and adherence to standardized practices.
Standardized Data Collection Forms: Standardized forms or questionnaires, including electronic forms, are used to collect data from participants. These forms have predefined fields, response options and consistent data formats across all participants or study sites, ensuring uniformity and simplifying data collection.
Quality Assurance and Monitoring: Regular quality assurance measures are implemented to monitor the data collection process, including electronic data collection. This involves periodic checks to ensure adherence to established procedures, site visits to verify protocol compliance and validation checks facilitated by electronic data collection systems.
Data Management Systems: Robust data management systems, such as electronic data capture (EDC) systems, are utilized to facilitate standardized data collection. These systems provide structured data entry interfaces, validation checks and secure storage, ensuring accuracy and reliability.
Data Cleaning and Validation: After data collection, including electronic data, thorough cleaning and validation are performed to identify and rectify errors or inconsistencies. This includes reviewing the data for missing values, outliers and logical inconsistencies facilitated by electronic data collection technologies.
Training and Quality Control
Ensuring that all individuals involved in data collection receive adequate training is crucial for maintaining accurate and complete data. Proper training equips data collectors with a thorough understanding of the study protocols, the data collection instruments and the significance of accurate data. It also helps them recognize and address any challenges or issues that may arise during the data collection process. Additionally, implementing regular quality control measures, such as data monitoring and audits, allows researchers to identify errors, inconsistencies or deviations from the established protocols. These quality control practices help maintain data integrity and enhance the overall reliability of the collected data.
Real-Time Data Entry and Validation
Performing real-time data entry into secure electronic systems, including electronic patient-reported outcomes (ePRO) and connected devices, offers several advantages for ensuring data accuracy. Immediate validation checks can be implemented, reducing errors and missing data. Range checks and logic checks can be applied during the data entry process, alerting data collectors to inconsistencies or inaccuracies. Timely correction or clarification can be made, enhancing data completeness. Real-time data entry and validation contribute to reliable and trustworthy clinical trial outcomes by improving data quality and integrity.
Participant Compliance and Monitoring
It is important to ensure participant compliance with data collection procedures to obtain accurate and reliable data in clinical trials. Clear instructions are provided to participants, outlining how and when to record the required information. Regular monitoring of participant compliance allows for timely intervention and identification of non-compliance or missing data. Besides, connected devices and wearable technology also assist in enhancing compliance and monitoring. These technologies enable continuous tracking of participant activities, providing real-time data and insights for better monitoring and assessment of compliance.
Data Quality Assurance
Ensuring data quality is a critical aspect of clinical data management. Regular quality assurance measures should be integrated throughout the data collection process to maintain the integrity and reliability of the data. This involves continuous monitoring of data quality, identifying and resolving any discrepancies or outliers and implementing data cleaning procedures. Techniques such as double data entry or data reconciliation can be employed to enhance accuracy and minimize errors. By proactively addressing data quality issues, researchers can mitigate the potential impact of erroneous or incomplete data on the study outcomes, ensuring the validity and robustness of the research findings.
Challenges During Data Collection
Collecting data in a clinical trial is a complex process that presents challenges such as data quality and accuracy, ethical and regulatory considerations, patient recruitment and retention, technology and infrastructure, etc. Researchers must anticipate these challenges and implement strategies to mitigate them effectively.
By addressing these challenges, researchers promote the collection of high-quality data, enhance the validity of study findings and ensure the reliability and credibility of the research. Moreover, navigating the complexities of data collection in clinical trials contributes to meaningful medical knowledge and patient care advancements.
Participant Recruitment and Retention
One of the significant challenges in clinical trials is successfully recruiting and retaining a sufficient number of eligible participants throughout the study duration. Low recruitment rates or high drop-out rates can have detrimental effects on the study’s validity and statistical power. Insufficient sample sizes may lead to biased results or an inability to draw reliable conclusions, both of which negatively impact the progression of a clinical trial. Researchers face the task of designing effective recruitment strategies, considering factors such as target population, eligibility criteria and recruitment timelines.
In such instances, electronic data collection devices, including apps on smartphones and wearable devices like smartwatches and fitness trackers, can greatly assist in maintaining data integrity and positively impact patient recruitment and retention. These technologies can remind patients when to collect and report data, automate data collection and minimize the burden on participants, thus increasing recruitment and retention rates. By leveraging electronic data collection devices, researchers can enhance participant engagement and ensure the integrity of the collected data.
Data Quality and Accuracy
Ensuring accurate and high-quality data in clinical trials is important, as human errors and challenges associated with self-reporting can compromise data integrity. Researchers can address these issues by implementing rigorous data quality control processes and standardized data collection procedures. Electronic data collection methods, such as electronic data capture (EDC) systems and mobile apps, can also help in improving data accuracy. These methods enable real-time data validation checks, reduce transcription errors and enhance data completeness. By leveraging electronic data collection, researchers can enhance the accuracy and reliability of the collected data in clinical trials.
Data Management and Organization
Effectively managing and organizing the large volumes of data generated during a clinical trial is crucial for data integrity and analysis. Researchers must implement robust data management systems and processes to handle data collection, storage and retrieval efficiently. Without proper organization, data can become disorganized, making it challenging to extract meaningful insights or conduct accurate analyses.
Implementing standardized data collection forms, establishing clear data coding systems and utilizing secure electronic data capture systems can help in streamlining data management processes. Electronic data capture systems can also assist in drawing insights and recommendations through effective data analysis methodologies. Additionally, adequate documentation and version control protocols contribute to data organization and facilitate data traceability throughout the study.
Technology and Infrastructure
Incorporating technology such as electronic data capture systems into data collection processes can offer advantages but also pose challenges. Technical issues, connectivity problems or complexities in the user interface may hinder the smooth flow of data collection. Researchers must ensure that technology is user-friendly and accessible to both participants and research staff. Adequate training and support should be provided to address any technological challenges or limitations. Regular monitoring and troubleshooting can help identify and resolve technical issues promptly, minimizing disruptions to the data collection process.
Ethical and Regulatory Considerations
Ethical and regulatory considerations play a vital role in clinical trial data management and collection. Researchers must adhere to established guidelines to protect the rights and welfare of participants and maintain the integrity of the data. This includes obtaining informed consent, ensuring privacy protection, and implementing robust data security measures. Navigating the complexities of ethical and regulatory requirements may require additional resources and time. Researchers should stay updated on relevant regulations and consult with ethics committees or regulatory authorities to ensure compliance throughout the data collection process.
Cross-Cultural and Language Considerations
In multicenter or multinational clinical trials, cross-cultural and language considerations pose unique challenges during data collection. Language barriers, cultural differences and diverse healthcare practices can impact the accuracy and reliability of the data. Researchers must account for these factors by implementing strategies to ensure effective communication and understanding among participants from different cultural backgrounds. This may involve translating study materials into multiple languages, employing trained interpreters and addressing cultural nuances in data collection instruments. By considering cross-cultural factors, researchers can enhance data quality, minimize biases and promote inclusivity in the study.
Quality control and assurance
Quality control and assurance are vital processes across industries to ensure that products and services meet required standards and customer expectations. Quality control involves systematically examining and testing products or processes to identify deviations from established specifications. It aims to prevent defects or errors and maintain consistency in production or service delivery. On the other hand, quality assurance focuses on managing and implementing quality control activities. It includes creating and enforcing quality standards, procedures and guidelines to achieve the desired level of quality.
These processes play a crucial role in enhancing customer satisfaction, reducing costs and improving overall efficiency. Implementing quality control measures allows organizations to identify and rectify defects or errors early, minimizing waste and rework. This, in turn, increases customer confidence and loyalty by providing consistently high-quality products or services.
Importance of Quality Control Measures in Clinical Data Management
Quality control measures play a vital role in ensuring the accuracy, integrity and reliability of clinical data throughout the data management process. By implementing these measures, researchers can generate high-quality data, enhance the integrity of study findings and contribute to meaningful advancements in healthcare. Effective quality control practices support patient safety, promote stakeholder confidence and facilitate evidence-based decision-making throughout the clinical research process.
Data Accuracy and Validity
Quality control measures help ensure the accuracy and validity of collected data. By implementing data validation checks, such as range or logic checks, inconsistencies or errors can be identified and rectified promptly. Electronic data collection aids in validation by detecting out-of-range or illogical instances. These checks highlight discrepancies in real time and enable prompt rectification. This improves the accuracy of human-written text, forming a reliable basis for analysis and informed decision-making. Accurate and valid data forms the foundation for reliable analysis and sound decision-making in clinical research.
Reliability of Study Findings
High-quality data obtained through effective quality control measures increases the reliability of study findings. By minimizing errors, biases and inaccuracies in the data, researchers can have confidence in the integrity of their results. Reliable findings contribute to the advancement of medical knowledge and inform evidence-based decision-making in healthcare.
Compliance With Regulatory Standards
Quality control measures help ensure compliance with regulatory standards and guidelines governing clinical research. Regulatory authorities require data to be collected, managed and reported in a standardized and accurate manner. Adhering to quality control practices ensures that the study meets regulatory requirements, enhancing the credibility and acceptance of the research.
Patient Safety and Well-Being
Quality control measures in data management have a direct impact on patient safety and well-being. Accurate and complete data enable researchers to identify adverse events, monitor patient responses and make timely interventions when necessary. Reliable data contributes to patient safety by supporting the evaluation of treatment efficacy and identifying potential risks or safety concerns.
Regulatory Requirements for Maintaining High-Quality Data
Clinical research data management requires compliance with regulatory requirements. Regulatory compliance ensures the integrity, reliability and ethical conduct of the study, thereby advancing medical knowledge, ensuring patient safety and facilitating the development of evidence-based practices. To meet regulatory standards for data quality and integrity, researchers must stay informed about relevant regulations, implement robust data management processes and engage in continuous quality improvement. By doing so, they can uphold the necessary standards and contribute to the progress of clinical research.
Good Clinical Practice (GCP) Guidelines
Good Clinical Practice (GCP) guidelines serve as an internationally recognized standard for conducting clinical trials with integrity and ensuring the protection of participants’ rights, safety and well-being. Adhering to GCP guidelines is crucial for maintaining high-quality data and upholding ethical standards in research. These guidelines provide principles and recommendations for data collection, documentation, verification and quality control, ensuring the reliability and integrity of the collected data. By following GCP guidelines, researchers demonstrate their commitment to conducting rigorous and trustworthy clinical trials, fostering trust among participants, regulatory authorities and the scientific community.
Informed Consent Process
The informed consent process is a fundamental requirement in clinical research that ensures participants are fully informed and voluntarily provide their data. It involves providing clear and comprehensive information about the study, including its purpose, procedures, potential risks, benefits and any alternatives. Participants must understand the nature of their participation and give their explicit consent before data collection begins. Adhering to proper informed consent procedures is essential for upholding ethical and legal standards, respecting participants’ autonomy and maintaining the integrity and validity of the collected data.
Protocol Adherence
Clinical research protocols outline the study design, objectives, methods and data collection procedures. Adhering to the protocol is critical for maintaining data quality, consistency and integrity. Regulatory requirements emphasize the importance of protocol compliance to ensure uniformity in data collection, minimize bias and facilitate reproducibility of the study results. Researchers must diligently follow the protocol’s instructions, document any deviations or amendments and justify them appropriately. By adhering to the protocol, researchers ensure that the data collected aligns with the study’s objectives, maintaining the credibility and validity of the research outcomes.
Data Traceability and Documentation
Regulatory authorities require comprehensive documentation of the entire data collection process in clinical trials, including data collection forms, case report forms, data dictionaries, source documents and any modifications made throughout the study.
This documentation ensures data traceability, facilitates data audits and supports the integrity and reliability of the collected data. Additionally, electronic data collection methods and repositories are crucial in enhancing documentation practices.
Maintaining clear and detailed documentation in electronic data repositories provides a centralized and accessible record of the data collection process. It enables researchers to track the origin and context of the data, ensures transparency for regulatory authorities and stakeholders and strengthens compliance with regulatory standards.
Data Privacy and Confidentiality
Protecting participants’ privacy and ensuring data confidentiality are paramount in clinical trials. Researchers must implement stringent data security measures, including secure storage, limited access and anonymization, to safeguard personal and sensitive information. Compliance with data protection regulations, such as the General Data Protection Regulation (GDPR), is essential to ensure the ethical and legal handling of participants’ data. Adhering to strict privacy and confidentiality protocols builds trust with participants and protects their rights, contributing to the overall integrity of the study.
Conclusion
In conclusion, effective data management plays a critical role in optimizing clinical trials. Managing large volumes of data poses challenges that can significantly impact trial efficiency and outcomes. However, by implementing robust data management strategies, researchers can ensure data integrity, enhance operational efficiency and expedite decision-making processes.
Moreover, effective data management allows for the maintenance of high-quality data, compliance with regulatory requirements and real-time access to accurate information, ultimately improving the reliability and validity of clinical trial results. It is essential for stakeholders involved in clinical trials to acknowledge the importance of data management and allocate appropriate resources and infrastructure to support it.
Future Developments in Clinical Data Management
Looking ahead, the field of clinical data management is poised for significant advancements. Rapid advancements in technology, particularly in the areas of artificial intelligence (AI) and machine learning (ML), hold great promise for transforming clinical trial data management.
AI and ML algorithms can assist in automating data collection, cleaning and analysis processes, reducing manual efforts and improving efficiency. These technologies can also facilitate the integration of data from diverse sources, such as electronic health records, wearable devices and patient-reported outcomes, providing a more comprehensive and holistic view of patients’ health status.
In addition to AI/ML, other future developments in clinical data management include advancements in data standards and interoperability. Efforts are being made to establish standardized data formats, coding systems and data exchange protocols to ensure seamless integration and interoperability between different clinical systems and databases. This will enable more efficient data sharing, collaboration and analysis across multiple trials and research institutions, fostering a more integrated and collective approach to clinical research data management.
Explore our comprehensive range of effective solutions designed to enhance patient care and accelerate medical advancements at Avantor. From clinical trials to diagnostic testing, our innovative services are tailored to meet the evolving needs of healthcare professionals.